Aktuelles
Informieren Sie sich über Angebote zu Studien mit innovativen Therapien und andere aktuelle Themen
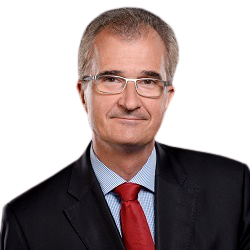
Herzlich Willkommen
auf der Website der Klinik für Psychiatrie und Psychotherapie des LMU Klinikums München.
Bei Fragen wenden Sie sich jederzeit gerne telefonisch unter 089/4400 55511 an unser Aufnahmeteam. Unsere Notaufnahme ist rund um die Uhr für Sie geöffnet.
Mit freundlichen Grüßen
Ihr Prof. Dr. Peter Falkai
Direktor der Klinik